
With advances in the power of technology and corresponding increases in data held online, it makes sense that government agencies would employ algorithmic decision-making systems (ADMs) that manage this data effectively and efficiently.
However, in a case study co-conducted by Professor Emerita Shirley Gregor on the way that an ADM was used in the Australian Government's Online Compliance Intervention – commonly referred to as 'Robodebt' – significant weaknesses were revealed that greatly impacted debt-collection recipients.
Shirley, from The Australian National University (ANU) College of Business and Economics (CBE), co-authored the paper titled Algorithmic decision-making and system destructiveness: A case of automatic debt recovery with colleagues Dr Tapani Rinta-Kahila, Dr Ida Asadi Someh, Professor Nicole Gillespie and Professor Marta Indulska, all from The University of Queensland Business School. The case study won two prestigious awards: The Stafford Beer Medal, 2023, for the best paper in the European Journal of Information Systems, and an award for being named in the top five 2023 Senior Scholar Best IS Publication Awards.
"Emerging technologies such as generative artificial intelligence are now widely available, and their weaknesses are often not well understood," says Shirley.
The Robodebt case showed how even relatively well-known technologies can lead to disastrous effects when inadequate software is coupled with an inability of top management to take action when defects become obvious.
Upon its implementation in 2016, Robodebt was expected to be a straightforward debt-collection process. The ADM they used would compare welfare recipients' records with income data from the Australian Taxation Office and pursue repayments based on its calculations.
The system, however, operated upon averages, which resulted in large miscalculations in amounts owed to the government, causing recipients considerable distress: a total of A$2 billion in debt notices were issued to 700,000 current or former welfare recipients.
The case study identified how ADMs restrict human agency, creating three limitations: minimised human oversight, reversing the onus of proof, and requiring citizens to self-service.
Minimising human oversight meant that all control of the system was managed by a central machine. This limited the checking of accuracy when the system made a debt estimation.
Furthermore, if recipients believed the debt estimation to be inaccurate, they themselves were required to prove the inaccuracy through acquiring old bank statements and pay slips from previous employers.
"Reversing the onus of proof is especially complicated when citizens are limited in their ability to obtain relevant information," says Shirley.
Even in a situation where a recipient required assistance with the process, Centrelink staff were instructed to redirect callers to their online portal.
"If self-service through online portals is encouraged, then user testing should be undertaken to ensure systems are accessible and user-friendly."
The mismanagement of the ADM would consequently cause debt-notice recipients and Centrelink staff considerable distress, and lead to a class action whereby the government agreed to a settlement of A$1.2 billion.
Despite this, ADMs have been, and remain to be effective vehicles for operative functioning in government agencies, when managed properly.
"A case in New Zealand with a child protection agency where children at risk might be removed from a family showed that, on balance, use of an algorithmic system gave better outcomes than non-use of the system," says Shirley.
"Still, human operators were able to override decisions on a case-by-case basis."
Reflecting on their case study, Shirley expresses that though ADMs and other information technology systems may be highly effective, it doesn't remove human responsibility from the equation.
Not having 'humans-in-the-loop' and relying exclusively on a machine to make decisions can lead to bias and unacceptable levels of error – an example of this is when the length of a prison sentence is set by a machine biased by race.
"Our society as a whole needs to work hard on ensuring these emerging technologies are developed and their use managed as well as possible. In any use of IT for decision making, thorough risk analysis should be undertaken."
The College is always keen to explore research collaborations with the public and private sector and to reconnect with alumni. Please get in touch if you would like to know more about partnering with us.
Featured expert
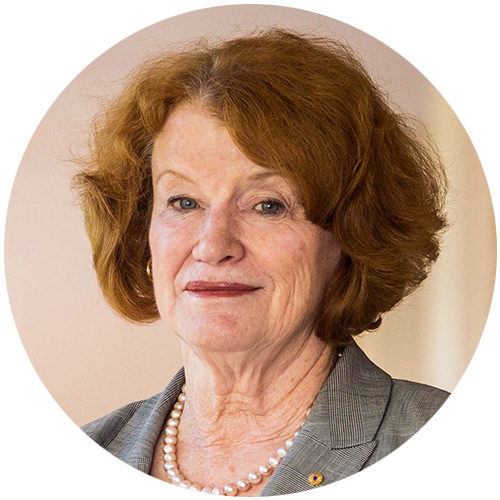
Professor Emerita Shirley Gregor
Shirley Gregor is Professor Emerita at ANU. Her research interests include artificial intelligence, human-computer interaction and the philosophy of science and technology. She is continuing with her long-term research in the area of ‘human-centred’ or ‘responsible’ AI, which has a focus on ethical considerations, transparency, and human wellbeing in the use of AI. She recently had a paper on Responsible AI and Journal Publishing appear in the Journal of the Association for Information Systems.